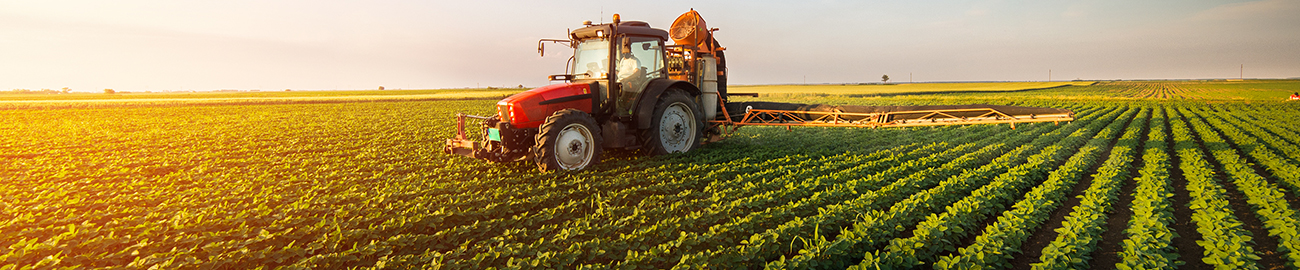
Improving projections of future land use and environmental impacts
Earth’s ecosystems are being pushed beyond safe limits in terms of freshwater use, biodiversity, and biogeochemical flows. According to researchers at the IIASA Ecosystem Services and Management Program (ESM), existing modeling tools need to be revisited to ensure that they are able to inform policies that can address this multi-faceted problem. To this end, ESM researchers refined the IIASA Global Biosphere Management Model (GLOBIOM) and started developing a flexible and scalable machine learning meta-model for the spatio-temporal downscaling of global crop model outputs.
As part of the Stimulating Innovation for Global monitoring of Agriculture (SIGMA) Horizon 2020 Project, ESM researchers embarked on a project to improve the cropland expansion and intensification process in the GLOBIOM agricultural and forest sector model. This included improving the downscaling functionality of the model to produce spatially explicit, reliable outputs on projected land-use change, and applying the model at the global scale to produce projections of cropland and major cropping systems for future scenarios in 2030 and 2050. In addition, the researchers developed methods to diagnose their impacts on greenhouse gas (GHG) emissions, reactive nitrogen flows, and terrestrial biodiversity.
As a first step, the ESM team improved the expansion and intensification modules of GLOBIOM. They collected new datasets to calibrate and set parameters for the new module functions, using a novel time series method to estimate crop and region specific price elasticities of area, quantity, and yield with a global coverage. Following this, they used the newly estimated elasticities to evaluate the behavior of the latest version of GLOBIOM. This diagnostic, along with additional data sources, and a novel global scale dataset of Environmental Policy Integrated Model (EPIC) crop model simulations (Hypercube), were used to improve the representation of cropland expansion and intensification in GLOBIOM. The team also used an econometric downscaling method for the land use and land use change results of GLOBIOM, where regional results are downscaled significantly. The method is capable of taking into account the uncertainty of existing land use maps, and provides valuable inference over the drivers of high-resolution land use change.
Based on this new downscaling module, the researchers were able to provide cropland projections for 2030 and 2050 along different Shared Socioeconomic Pathways (SSPs) and Representative Concentration Pathways (RCPs).
To assess the environmental impacts of these high resolution land use changes, the team also developed novel methods to translate land cover and land use changes into habitat changes, species richness, and loss of threatened endemic species (in collaboration with ETH Zurich), by integrating a new dataset of estimates of scale water and nitrogen balances using the EPIC crop model. Preliminary results indicate, for example, that by 2050, significant intensification of cropland will increase the amplitude of nitrogen input to cropland and losses to the environment by more than 50%. Future changes in cropland extent will generate a moderate (less than 10%), but robust decrease in the average global species richness and loss of threatened endemic species richness. This implies that without mitigation efforts, the future expansion of cropland will generate additional GHG emissions. Future cropland extension could also amplify the effect of projected increase in cropland intensity in some scenarios, while increased climate change mitigation reduces cropland expansion and could lead to co-benefits for biodiversity.
Apart from the refinement of GLOBIOM, the Agro-Environmental Systems team at ESM also started developing a flexible and resource-efficient machine learning (ML) meta-model that would allow for spatio-temporal downscaling of crop model outputs at a high spatial resolution.
The IIASA-EPIC global gridded crop modeling (GGCM) framework, produces outputs at spatial resolutions that are often too comprehensive for use in regional and local decision making. The EPIC Hypercube dataset for example, provides monthly to annual data on global crop production, crop intensification responses, and associated environmental externalities. In 2017, in a collaboration with the International Maize and Wheat Improvement Center (CIMMYT), the team implemented a number of ML algorithms, namely gradient boosting and random forests, to downscale global EPIC Hypercube simulations of maize yield potential to a finer resolution at the extent of Mexico (project ColClim).
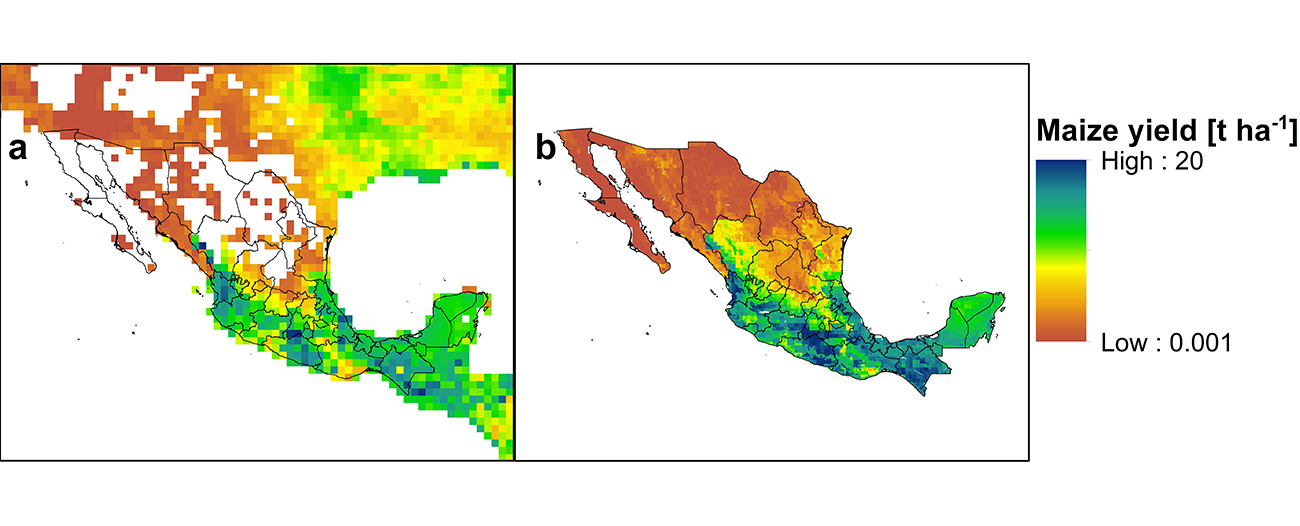
Examples of rain-fed maize yields for the year 2000 from (a) global IIASA-EPIC Hypercube at 0.5×0.5 arc-deg, and (b) corresponding ML predictions for Mexico at 1×1 km.
The ML model was tested for downscaling selected agricultural externalities, in addition to crop yields. Since additional data dimensions and environmental predictors are needed for developing a flexible ML meta-model, the team collaborates with the existing GGCM community and its ongoing activities, including the Agricultural Model Intercomparison and Improvement Project (AgMIP) and the Inter-Sectoral Impact Model Intercomparison Project (ISI-MIP), to facilitate this requirement [1][2]. The ML meta-model provides the means to inform decision-making processes at regional and sub-regional scales
These methodological improvements allow comprehensive quantitative assessments of future land use change and related policies, and will increase the ability of researchers to cooperate both within and outside of IIASA. This work was presented at conferences [3][4] and has led to several publications [5][6][7]. It is also currently contributing to the Intergovernmental Science-Policy Platform on Biodiversity and Ecosystem Services (IPBES) process and generating new scenarios, which are expected to lead to high policy impact in 2018.
References
[1] Müller C, Elliott J, Chryssanthacopoulos J, Arneth A, Balkovic J, Ciais P, Deryng D, Folberth C, et al. (2017). Global Gridded Crop Model evaluation: benchmarking, skills, deficiencies and implications. Geoscientific Model Development Discussions 10: 1403-1422
[2] Rosenzweig C, Elliott J, Deryng D, Ruane AC, Mueller C, Arneth A, Boote KJ, Folberth C, et al. (2014). Assessing agricultural risks of climate change in the 21st century in a global gridded crop model intercomparison. Proceedings of the National Academy of Sciences 111 (9): 3268–73.
[3] Krisztin T, Havlik P, Leclère D, & Moreau I (2015). Global High-resolution Land-use Change Projections: A Bayesian Multinomial Logit Downscaling Approach Incorporating Model Uncertainty and Spatial Effects. In: Systems Analysis 2015 – A Conference in Celebration of Howard Raiffa, 11–13 November 2015, Laxenburg, Austria. 9th International Conference on Computational and Financial Econometrics (CFE) 2015, 12–14 December 2015, London, UK.
[4] Leclère et al. (2017). Hotspots of future land use change impacts on terrestrial biodiversity under global mitigation scenarios. 2017 World Impacts conference, 10–13 October 2017, Potsdam, Germany.
[5] Prestele R, Alexander P, Rounsevell,M, Arneth A, Calvin K, Doelman J, Eitelberg D, Engström K, et al. (2016). Hotspots of uncertainty in land use and land cover change projections: a global scale model comparison. Global Change Biology 22(12): 3967–3983.
[6] Byers E, Gidden M, Burek P, Ebi K, Greve P, Havlík P, Johnson N, Kahil T, Krey V, Langan S, Leclère D, Obersteiner M, Palazzo A, Pachauri S, Parkinson S, Rao N, Rogelj J, Satoh Y, Wada Y, Willaarts B, Riahi K (2018). Global exposure and vulnerability to multi-sector climate change hotspots. Environmental Research Letters, under revision.
[7] Alexander P, Prestele R, Verburg PH, Arneth A, Baranzelli C, Batista e Silva F, Brown C, Butler A, et al. (2017). Assessing uncertainties in land cover projections. Global Change Biology 23: 767–781.
Collaborators
- IIASA – Water Program, Energy Program, Air Quality and Greenhouse Gases Program
- ETH Zurich, Switzerland
- The Intergovernmental Science-Policy Platform on Biodiversity and Ecosystem Services (IPBES), Bonn, Germany
- World Wildlife Fund (WWF)
- Wageningen University, Netherlands
- National Institute for Environmental Studies (NIES), Japan
- Tokyo University, Japan
- UN Environment World Conservation Monitoring Centre (UNEP-WCMC), Cambridge, UK
- The Commonwealth Scientific and Industrial Research Organization (CSIRO), Canberra, Australia
- Potsdam Institute for Climate Impact Research (PIK), Germany
- Centre for Hydrology and Ecology (CEH), UK
- New York University, USA
- International Maize and Wheat Improvement Center (CIMMYT), Mexico
Further information
Other highlights
Top image © Fotokostic | Shutterstock
You must be logged in to post a comment.